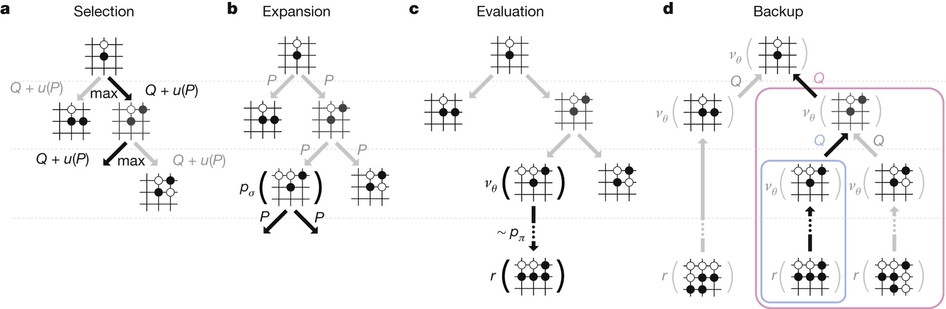
Deepmind now introduces AlphaGo as “the first computer program to ever beat a professional player at the game of go”. Powered by 1202 central processing units (CPUs) and 176 graphic processing units (GPUs), just with its hardware, AlphaGo ranks among the top 500 high-end computers in the world. However, what makes AlphaGo even more special and powerful, to such an extent to be deemed infallible is the deep learning technology.
AlphaGo takes advantage two neural networks, so called “value networks” and “policy networks”, which are used to evaluate board positions and select moves, respectively. The neural networks are continuously trained through 3 main stages. In the first stage named supervised learning of policy networks, supervised learning policy network outputs the probability density distribution for all legitimate moves of a player, predicting the expert moves in the game. Next, policy networks are further ameliorated by policy gradient reinforcement learning. Reinforcement learning policy network starts with the same initial conditions with the supervised learning policy network. Then it is improved by winning more games against the previous iteration of policy networks. Simply put, games of self-play are played between the current policy network and a randomly selected version of pervious policy network, creating a new data set. The final stage is reinforcement learning of value networks. Through evaluation of the board positions, the value network outputs a single prediction about whether the current player wins in positions from the self-play data set. Monte Carlo tree search algorithm works in concert with the two networks of AlphaGo. Each simulation of the Monte Carlo tree search algorithm begins as the simulation picks a node and expands the node. Then, the expanded node is evaluated by using the value network and running a demonstration to the end of the game, computing the winner of the game at each node. Through the combination of neural networks and tree search, AlphaGo was able to make a historical achievement in AI.
저작권자 © 포항공대신문 무단전재 및 재배포 금지